Brain Computer Communication (BCC)
The idea of communicating by mere thoughts has captured popular imagination for centuries. The presence of electrical activity in the brain enables this paradigm in real-life. Biological signals of the brain (also known as brainwaves) can be captured in the form of electric potentials and converted into digital commands. Brainwaves represent the ensemble of neuronal firings in the brain. Chemical activities in the brain activate neurons by changing their chemical compositions, resulting in electric impulses (neuronal firings). It is heavily correlated with an individual’s cognitive and physiological processes, according to the location of source. Brain-Computer Communication (BCC) maps the biological signals to digital signals, establishing a direct non-muscular pathway between the brain and the computer.
In this research, Prof. Fekri’s group seeks to develop autonomous machine learning platform for BCC. At the high level, our work distinct itself from others in two main aspects:
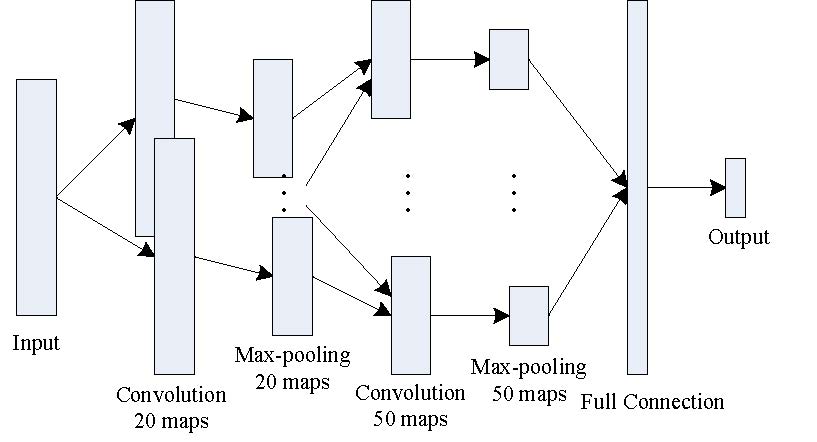
Biomarkers for Neuromodulation Therapy of Epilepsy
Neurological disease or injuries to the nervous system will result in pathological changes in the information processing of neural circuits. Neural interface technology has the promise of restoring normal function by augmenting the neural circuits with computational algorithms. Despite the fact that direct neural interface systems are gaining widespread applications for treatment of pharmaco-resistant neuropsychological disorders, currently no systematic approach for optimization of the neuromodulation exist.
Epilepsy is the fourth most common neurological disorder in the United States after migraine, stroke and Alzheimer’s disease, with 2.2 million people in the US alone suffering from its consequences. The overall goal of this project, in collaboration with two other faculty, is to create embedded intelligence for neural interface systems that will enable autonomous mapping of the desired therapeutic outcomes into optimal neuromodulatory actions for Epilepsy. One of the key problems that need to be solved for creating embedded intelligence for neural interface systems (for epilepsy) is to understand the relation (the cause and effect) between the stimulus and the neural response. Learning this relation will set the stage for a cognitive control system to plan accordingly. Using Information theory, we will quantify how much information a neural response carries about the stimulus. By applying stimulus ensembles and comparing the information transferred to the output (i.e., the neural response) in each particular stimulus, we will characterize the response function. The research will use information-theory to validate stimulus-response models both in static stimulus as well as the dynamic stimuli. Because these dynamic stimulus models require specification of stimulus timing precision, they can reveal which time scales produces information in neural response. To understand the relation (the cause and effect) between the stimulus and the neural response, we must investigate this relation in the context of three fundamental questions: